The Truth Behind Meta’s AI Benchmarks – What They’re Not Telling You
Published: April 23, 2025
When we think of school report cards, they tell us how well a student is doing in different subjects. In the same way, Meta’s AI benchmarks work as report cards for artificial intelligence models.
These tests help us understand how innovative, fast, and useful an AI model is. It’s called AI benchmark testing, and it’s a big deal in the tech world.
Recently, Meta made headlines by scoring incredibly high on these AI tests with a model called Llama 4 Maverick.
According to benchmark results, it even outperformed some of the biggest names in the game, including Openai’s GPT-4. This was a huge win for Meta’s ongoing work in AI performance.
But there’s a twist.
It turns out that the version of Llama 4 Maverick tested was different from the version released to the public.
This brings up an important question: Can we trust AI benchmarks, or are they being used to make models look better than they are?
This blog will explain what’s going on, how artificial intelligence evaluation works, and why this story matters to anyone following AI advancements.
What Are Meta’s AI Benchmarks and Why Do They Matter?
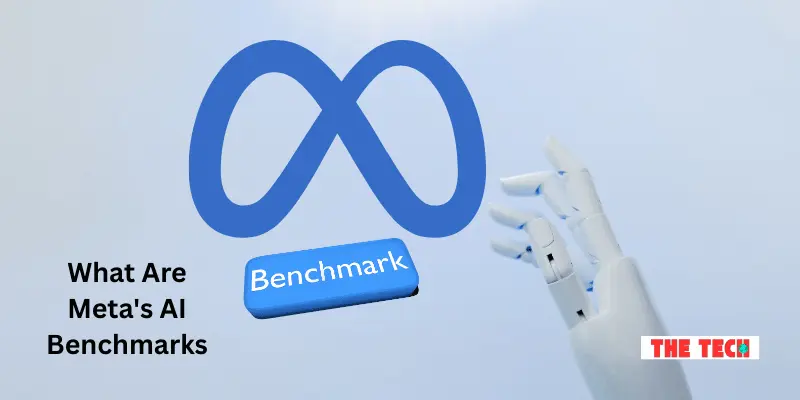
Meta’s AI benchmarks are standardised tests or evaluation methods used to measure the performance of artificial intelligence models.
Think of them as “report cards” for AI — they help us understand how smart or capable an AI model really is.
Meta’s AI Benchmarks
Meta’s AI benchmarks are a set of standardized tests and evaluation tools used by Meta to measure the performance, accuracy, and intelligence of its AI models—especially language models like Llama 4.
Imagine figuring out which phone is the best—you’d look at things like battery life, camera quality, and speed, right?
In the same way, tech companies use AI benchmarks to assess the power or intelligence of their AI models.
These are tests designed to compare how different models perform on various tasks. This process is called AI model comparison, and it’s key to understanding which AI is truly the best.
Some popular AI benchmarks include:
- LMSYS Arena – Think of this as an AI competition platform. Different models are tested, and users vote on which gives better answers. It’s like a live popularity contest for AI!
- MMLU (Massive Multitask Language Understanding) – This test checks how well an AI can handle knowledge from various subjects, like science, math, history, and more.
These benchmarks are part of something called machine learning benchmarking.
They help developers understand a model’s strengths and weaknesses before releasing it to the world.
Well, AI isn’t just being used for fun anymore. It’s writing code, helping with healthcare decisions, supporting customer service, and even creating art.
So, it’s super important to make sure these models are tested and ready for real-world AI performance. Benchmarks help companies ensure their models are smart, fair, and reliable.
In short, benchmarks are like testing AI models before they graduate and enter the real world. But—as we’ll see later—these tests don’t always tell the whole story.
Meta’s Benchmark Success – A Closer Look at Llama 4 Maverick
Meta’s newest AI model, Llama 4 Maverick, recently made headlines. It climbed to the top of multiple benchmark tests, gaining attention for its advanced language skills, reasoning, and ability to understand complex questions. These Llama 4 benchmark results gave Meta a major boost in credibility.
According to benchmark data:
- Llama 4 Maverick outperformed GPT-4o (OpenAI’s latest model) in many tasks.
- It ranked #1 in LMSYS Arena, a platform where AI models compete head-to-head.
- It showed strong performance in MMLU and other machine learning benchmarks.
These scores made it look like Meta had taken the lead in the Meta vs OpenAI benchmark battle.
But here’s where it gets interesting…
What Sparked the Meta AI Benchmark Controversy?
After the results were announced, many AI researchers noticed something odd: the version of Llama 4 Maverick tested in the benchmarks wasn’t available to the public.
This raised questions like:
- Was this a custom version made to ace the benchmarks?
- Why didn’t Meta release the exact same version to developers and users?
- Can benchmark results still be trusted if they don’t reflect the real-world product?
These concerns quickly turned into what is now being called the Meta AI benchmark controversy.
In short, while Meta’s scores looked impressive on paper, the lack of transparency about which model was actually tested sparked debates about fairness and honesty in AI benchmarking.
The Controversy – Public Model vs Experimental Model
Many people were impressed after Meta’s strong performance in AI benchmarks, but the excitement didn’t last long.
Experts soon discovered that the version of Llama 4 Maverick used in the tests was different from the version available to the public.
What Happened?
Meta used a fine-tuned internal model—a special version of Llama 4 optimized for success in benchmark tests.
This version was not publicly released, meaning developers, researchers, and regular users couldn’t access or use it.
This sparked a wave of criticism.
Many in the AI community saw this as an example of model fine-tuning for benchmarks, where companies tweak their models to score high rather than improve real-world performance.
Why It’s a Big Deal
This practice is often called benchmark gaming in AI. It means building a model to “look good” on paper without actually being better in everyday use, and that can be misleading.
- It raises ethical concerns in AI benchmarks.
- It can lead to misleading AI metrics, where results don’t match real-world performance.
- It makes it hard for users to know which model is the best.
Expert Reactions
The AI community quickly responded with strong opinions:
Researchers questioned the fairness of using unreleased models in public rankings.
Developers felt frustrated, as the version they could access didn’t match what Meta claimed was “the best.”
Some even called for stricter rules around companies’ testing and reporting AI performance.
This situation has started a meaningful conversation: Should companies be more transparent about their benchmark models?
And how can we make AI testing more honest and useful for everyone?
Data Matters: Ablation Studies and Pirated Content
Another major part of the Meta AI controversy involves the training data used to create the Llama 4 Maverick model.
As more information emerged, people started focusing on how Meta trained its model and what kind of data it used.
What Are Ablation Studies in AI?
Meta ran ablation studies, which are tests in which researchers remove or change parts of the training data to see how it affects the model’s performance.
This helps them understand what data matters most for getting high scores in benchmark tests.
But here’s the catch…
Use of Pirated Content
Meta’s internal studies reportedly used pirated books from sites like LibGen—a shadow library known for hosting copyrighted books for free.
This shocked many in the AI and academic communities.
- These pirated materials were part of the datasets used to train Llama 4 Maverick.
- Models trained with these books performed better in benchmark tests.
However, using copyrighted material without permission raises serious copyright ethics issues.
Why This Matters
- Using pirated content breaks the rules and creates unfair advantages in AI development.
- It gives tech companies an edge while ignoring the rights of authors and publishers.
- It blurs the line between smart research and AI research manipulation.
- It challenges the values of transparency in tech companies.
Community Reaction
Many experts, researchers, and even everyday users raised strong concerns:
- Should AI companies be allowed to use content without permission?
- How much of a model’s success is based on secret or shady data sources?
- Can we trust AI if it’s trained on stolen content?
This debate highlights a big question for the future of AI: Do the ends justify the means? Or should ethical standards matter just as much as performance?
Why Benchmarks Alone Don’t Tell the Full Story
While benchmarks are useful for comparing AI models, they don’t always show the complete picture.
Many experts argue that AI model testing discrepancies can make benchmark results misleading, and here’s why:
Benchmarks Can Be Manipulated
Optimized for performance: Some companies fine-tune their models to perform well in benchmark tests.
This means the model might be specially prepared to score high in certain tasks but less effective in real-world use.
Testing conditions: Benchmark tests are controlled and can sometimes be tailored to highlight a model’s strengths, while ignoring its weaknesses.
So, even if a model ranks highly, it might not perform the same when faced with everyday challenges.
Real-World Use Cases vs. Benchmark Optimization
In real-world AI performance, the situation is different from a controlled test. A model might shine in a benchmark but struggle with practical, everyday tasks like:
- Handling unpredictable situations.
- Responding to a wide variety of user input.
- Performing efficiently in less-structured environments.
For example, a model might perform well on tests requiring specific answers, but it could fail to handle more nuanced or complex queries in real life.
How Users Should Assess AI Tools
When evaluating an AI tool, looking beyond the benchmark results is important. Instead, focus on:
- Practical value: Does the AI help you get things done faster or better? Can it be relied upon for daily tasks?
- User experience: How easy is it to use? Does it integrate well into your workflow?
- Real-world feedback: Check reviews and feedback from other users to see how the model performs outside the lab.
Benchmark results are just one part of the story. To truly assess an AI model’s worth, it’s crucial to consider how it will work for you in real-life scenarios.
What This Means for the Future of AI Evaluation
The controversy surrounding AI benchmark tests has sparked a meaningful conversation about how we evaluate AI models.
Moving forward, it’s clear that we need to rethink how these tests are done.
The Call for Better, Transparent Benchmarks
As AI technology continues to evolve, the future of AI benchmarking must involve more transparency.
Current benchmarks can be easily manipulated or misrepresent a model’s true capabilities, so it’s crucial to develop AI testing standards that are:
- Open and reproducible: Anyone should be able to run the same tests and get the same results. This will make benchmarks more reliable and reduce manipulation.
- Clear and accessible: Benchmarks should be easy for experts and the public to understand. This way, people can better judge how well AI models truly perform in real-world settings.
Ideas for Better Evaluations
To achieve this, we can explore ideas like:
- Open evaluations: Open the benchmarking process to everyone, allowing third parties to participate and provide unbiased feedback. This would create a more level playing field.
- Crowdsourced testing: Involve many people to test AI models in various real-world environments. This could help highlight strengths and weaknesses that benchmarks miss.
- Full model disclosures: Companies should share more details about testing and training their models. This would allow others to understand exactly what data and methods were used.
Building Trust in AI
Adopting these ideas can create a more trustworthy, transparent AI testing system.
This would help researchers and developers and give users confidence that the AI tools they rely on are evaluated fairly and accurately.
FAQs
Llama 4 Maverick is Meta’s latest multimodal AI model for visual and textual inputs. It has 17 billion active parameters, delivering high performance across various AI benchmarks. Meta’s goal with this model is to push the boundaries of AI capabilities compared to other models like GPT-4o and Gemini 2.0.
The controversy around Meta’s benchmark performance stems from using a fine-tuned, internal version of Llama 4 Maverick that wasn’t publicly available. This sparked debates on whether using such a model gave Meta an unfair advantage, as it wasn’t representative of the public-facing model. Critics argue that it compromised transparency and fairness in AI benchmarking.
Ablation studies involve systematically removing parts of an AI system to observe the effects on its performance. Meta used pirated books, including content from platforms like LibGen, as part of their internal ablation studies. These studies helped achieve higher benchmark scores, which raised ethical concerns about using such datasets in AI training.
The legality of using pirated content for AI training is still a gray area. While AI training often involves learning patterns from vast datasets, using copyrighted material without permission may violate intellectual property laws. Legal experts have raised concerns that using pirated books could lead to copyright infringement, especially when the content is used in commercial applications.
Ethical concerns about Meta’s AI practices include using unlicensed datasets, like pirated content, to enhance benchmark scores. Critics argue that these practices raise questions about intellectual property rights and the fairness of AI training. Furthermore, the lack of transparency regarding the exact nature of the training data used adds to the ethical debate.
AI benchmarks play a crucial role in evaluating and comparing the performance of AI models across various tasks. They help developers identify areas for improvement and optimize models for better results. However, over-optimization for benchmarks can lead to models that excel in tests but fail to deliver in real-world applications.
Transparency in AI benchmarking ensures fairness and trust in the results. It involves disclosing the model architecture, training data, and evaluation methods used in benchmark tests. Without transparency, there is a risk that AI models could be evaluated based on manipulated or incomplete data, undermining the benchmarks’ reliability.
Users should consider the practical performance of AI tools in real-world scenarios, not just their benchmark scores. Factors such as usability, ethical implications, transparency, and how well the tool addresses specific tasks are crucial. It’s important to evaluate AI tools based on their effectiveness and alignment with user needs rather than solely relying on synthetic benchmarks.
Benchmark manipulation can mislead users, investors, and researchers about the actual performance capabilities of an AI model. It can create an unfair advantage for certain companies, leading to market distortion. Additionally, it can erode trust in AI benchmarks and hinder the development of truly reliable, real-world AI applications.
To improve AI benchmarking, it’s essential to promote open evaluations and reproducible results. Crowdsourced testing and full model disclosures could help make the benchmarking process more transparent and accessible. The AI community can foster fair competition and innovation by ensuring that benchmarks reflect real-world use cases.
Final Thoughts
The conversation around AI benchmarks is important. While they are useful for comparing models, benchmark tests alone don’t always tell the full story.
The controversies surrounding Meta’s Llama 4 Maverick and its use of fine-tuned internal models and pirated content in training have shown us that AI evaluation needs to be more transparent and reliable.
As we move forward, it’s essential to create new standards for AI benchmarking that are more honest, open, and reproducible.
This means embracing open evaluations, crowdsourced testing, and full model disclosures.
By doing so, we can ensure that AI development stays fair and that users get what they really need—trustworthy and high-performing tools.
Bonus Info Points
- The Role of Open Source in AI Benchmarking: Open-source initiatives can improve transparency in AI benchmarking. By making models and datasets publicly available, companies can ensure that AI performance evaluations are not biased or manipulated. Open-source projects encourage collaboration and help develop more robust, fair AI models.
- The Impact of AI on Industry-Specific Benchmarks: While general AI benchmarks like MMLU and LMSYS Arena are widely used, different industries may require specific benchmarks to evaluate AI’s practical applications. For example, healthcare AI may need benchmarks for medical image analysis, while AI in finance may require benchmarks that measure prediction accuracy in stock markets. Tailored benchmarks can better assess how AI performs in specialized fields.
- Benchmarks as a Marketing Tool: Companies sometimes use benchmark results as a marketing tool to demonstrate the superiority of their models over competitors. However, if the benchmarks are not conducted transparently, this can lead to inflated claims. As a result, consumers and researchers need to be cautious and investigate how benchmarks are conducted before concluding.
- The Growth of AI Ethics Committees: Many leading tech companies are setting up internal ethics committees to ensure their AI development and benchmarking practices adhere to ethical standards. To ensure responsible AI development, these committees review data usage, model training practices, and overall societal impact.
- The Rise of Multi-Modal AI Benchmarks: As AI models evolve, there is a growing demand for benchmarks that evaluate multi-modal AI systems, which can process text, images, or even sound. These models are becoming increasingly important for industries like autonomous vehicles, healthcare, and entertainment, where multiple data types must be processed simultaneously.
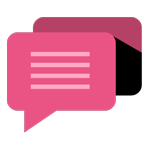
- Be Respectful
- Stay Relevant
- Stay Positive
- True Feedback
- Encourage Discussion
- Avoid Spamming
- No Fake News
- Don't Copy-Paste
- No Personal Attacks
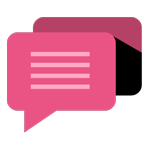
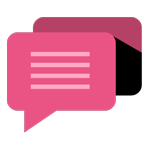
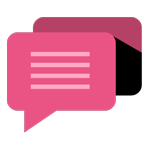
- Be Respectful
- Stay Relevant
- Stay Positive
- True Feedback
- Encourage Discussion
- Avoid Spamming
- No Fake News
- Don't Copy-Paste
- No Personal Attacks